Table of Contents
Artificial intelligence and machine learning are on a rise, and healthcare is among the industries where AI/ML can drive changes to drastically reinvent approaches to diagnostics, patient care, hospital management, etc.
Artificial intelligence is always tied to data, and for data-intense sources like medical images, it can power up a new level of analytical depth and help radiologists process images more efficiently and precisely than ever.
The workload of radiologists increases exponentially: in some cases, average radiologists need to read a medical image within 3-4 seconds to be able to finish the amount of work they have for an 8-hour day. Of course, such an intensity won’t reflect positively on healthcare professionals’ mental state and concentration, which eventually leads to low diagnostic accuracy, additional stress for patients, and fast burnout for healthcare specialists.
Considering these conditions, medical imaging machine learning is a key to relieving radiologists from excessive workload, but the true potential of these technologies goes far beyond and allows detecting abnormalities in medical images that the human eye must miss or misinterpret.
Let’s contemplate some practical cases of artificial intelligence and image recognition software being applied to the field of medical image analysis.
Brain Tumor
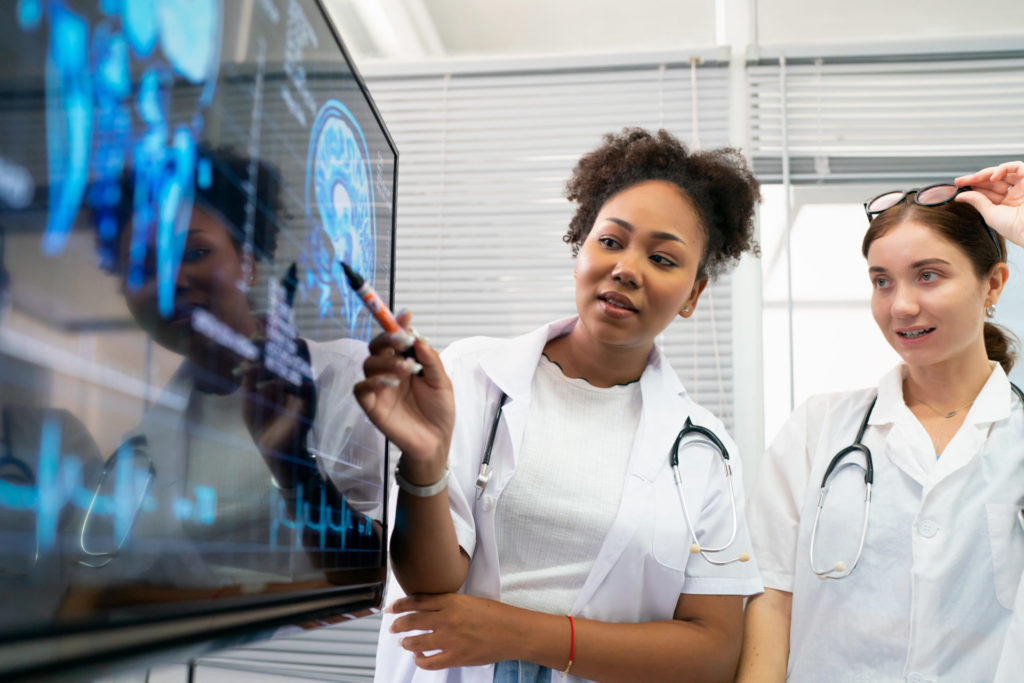
Neurological Diseases
Breast Cancer
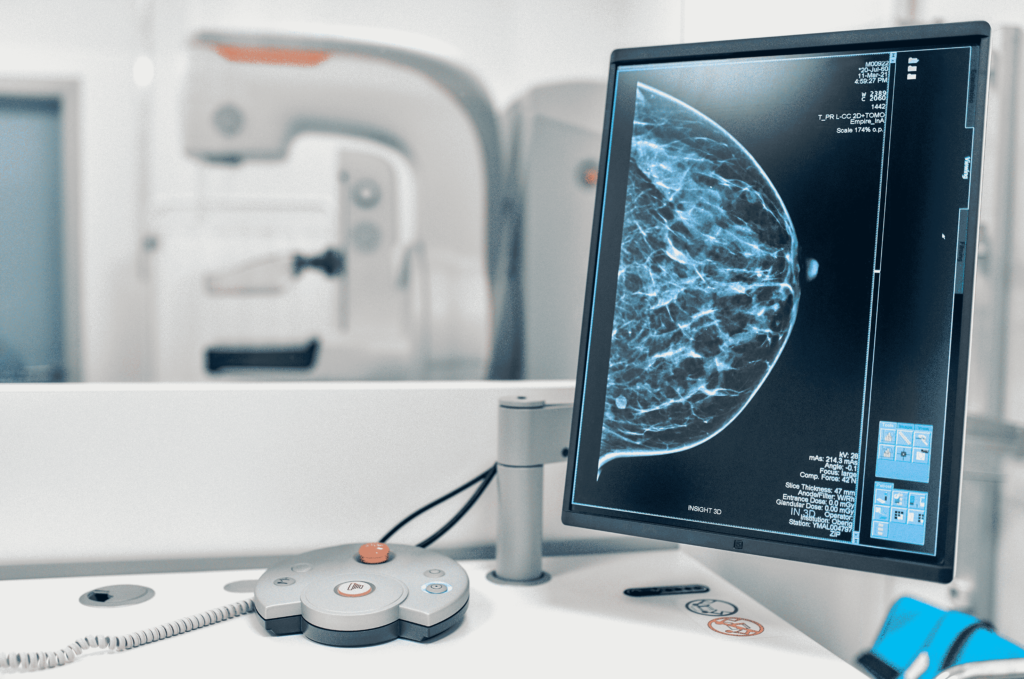
Cardiovascular Issues
Musculoskeletal Injuries
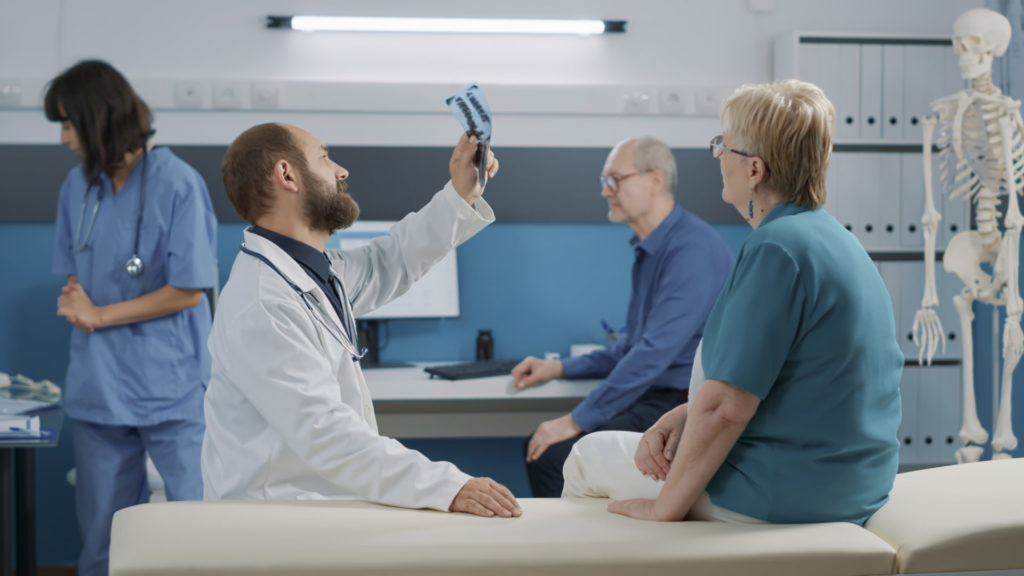
AI can contribute a lot to the detection of musculoskeletal issues, namely bone fractures. There are several reasons why fractures might be unnoticed by clinicians (overload that increases the chance of error, prioritization of other clinical concerns like internal bleeding or organ injury, lack of radiologists in a facility, etc.).
Whatever the case is, artificial intelligence can aid healthcare professionals and improve the fracture diagnosis success rate or act as a “second reader” to ensure accuracy. However, as with many other AI-powered medical imaging tools, it is still in the early stages of its development and demands improvements before being implemented into the clinical environment for the actual process of detecting fractures.
At the same time, AI can be used to analyze medical imaging data to detect other musculoskeletal injuries and issues, like identifying the problems with joint replacement devices, more efficient assessment of rotator cuff tears, etc.
Second Opinion
While the majority of artificial intelligence algorithms might not still be sufficiently trained to be the only basis for decision-making, AI for medical imaging can be successfully used as a “second reader” to provide a second opinion for a certain case or to run a cross-validation of clinicians’ diagnosis if there are any doubts. With the relevant algorithms, it can work for any type of medical imaging (MRI, CT, ultrasound, X-ray images) and become a powerful aid to radiologists who suffer from excessive workloads.
In 2020, in the middle of the COVID-19 pandemic, US researchers created an AI algorithm that allows the detection of COVID by analyzing CT images of patient lungs. The value of this study is especially high: at the moment, CT has not been the most common method of detecting COVID, with genetic exams prevailing. While labs have been overwhelmed, CT could become another option for diagnosis, which would increase the percentage of early diagnosis and timely isolation of infected patients.
In this case, AI will strengthen the diagnostic capacities of clinicians by being able to detect the disease during the early course of the illness or by discovering non-specific findings.
Radiation Dosage Reduction
The Main Challenges Behind Artificial Intelligence and Machine Learning in Medical Imaging
AI in medical imaging is a hot topic, however, there are still significant challenges that scientists, AI developers, and medical professionals should address to achieve success in the field.
Generalizability of Models
Deep learning models, widely used for medical image analysis, are trained on certain data pieces to predict outcomes when being fed raw data. But what if the data you are going to feed to the model differs from the original pieces, e.g., if you decide to use data for patients from another geographical region? Would you be able to trust the algorithm, will it be able to make the right conclusions?
There is always a chance that an algorithm will show lower accuracy on a dataset different from the one that was used to study the algorithm, and it might be the biggest challenge that stops AI-based medical imaging tools from clinical implementation.
Data Acquisition
To create a precise solution, developers need a lot of representative data to train the algorithm. But it is pretty hard to acquire enough data, as medical institutions don’t want to share data easily. And of course, there is also an important question of regulations and privacy that complicates data acquisition.
Lack of Infrastructure in Hospitals
There is a lack of clinical trials, and the main reason is that the majority of hospitals don’t have the computational infrastructure to track and evaluate the performance of AI algorithms, which makes it pretty hard to run clinical trials and check the deep learning performance in a clinical environment.
In Conclusion
The implementation of artificial intelligence for medical imaging has a lot of potential, no wonder it is arousing the interest of researchers. And while some of the solutions are already used in certain medical institutions, AI-based tools are still in the early stages of their development, and their real mass use is lying ahead of us.
Still, hospitals and other medical institutions can benefit from AI capacities right now and improve the lives of clinicians and patients. Image recognition can lower the probability of human errors in medical records and help you to process some cases of medical images.
AI-based Solutions for Your Medical Institution
Enhance the performance of your team with the help of artificial intelligence
Contact usThe most common type of machine learning used for medical imaging purposes is deep learning.
Machine learning is used to recognize patterns: the algorithm computes the image features, that identify the best combination of these features to classify the image or to create some metrics related to a certain image region.