Table of Contents
The times when a simple bank statement could encapsulate one’s financial activity is a thing of the past. Today’s client profile is more complex and nuanced, covering transactional data and a diverse range of details, including personal information, online search behaviours, shopping preferences, media consumption patterns, social network activities, frequency and locations of travel, and broader areas of interest. This variety of data paints a far more multi-dimensional picture of the client’s financial life.
Financial institutions proactively use this wealth of information by blending client knowledge with data gathered from various sources.
Such a mix of information enables them to understand specific individuals and groups of customers better. Equipped with this insight, they can tailor their services and offers more effectively, improving personalisation levels.
What is big data?
What exactly is big data, and where does it originate? In a nutshell, big data refers to vast and ever-expanding collections of data sourced from numerous channels, both in type and structure. This data, often complex and bulky in its raw form, poses considerable challenges in its management.
A key attribute of big data is its continuous growth in both volume and intricacy.
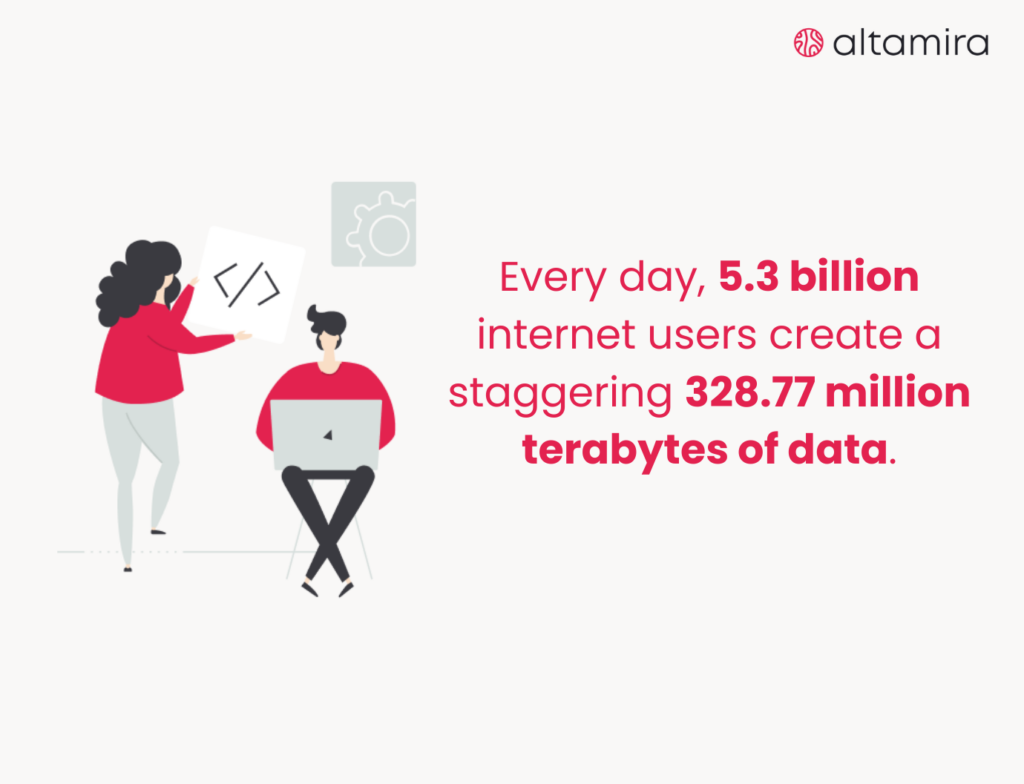
The catalytic influence of big data is undeniable. It is the engine that improves operational efficiency, sculpting a new age of personalized financial services. Oleksandr Budnik, the Chief Technology Officer at Altamira, brings his unique and authoritative perspective:
“Based on industry experiences, the benefits and importance of Big Data Analytics in Fintech include:
- Improved Decision Making
- Fraud Detection and Prevention
- Enhanced Customer Experience
- Risk Management
- Cost Reduction
- Regulatory Compliance
- Innovative Products and Services
Moreover, the fusion of Big Data with Machine Learning capabilities marks a significant opportunity. This combination evolves into a potent tool, substantially elevating business performance.
Machine Learning algorithms can delve into complex, large datasets to reveal hidden patterns, trends, and correlations, leading to more refined predictive models and intelligent decision-making.
For example, ML enhances algorithmic trading, revolutionizes credit scoring, and personalizes financial advice. The integration of Big Data with ML not only furnishes a competitive advantage but also fosters new pathways for innovation and business expansion in fintech.
This relationship between Big Data and ML is crucial in propelling the future of financial technologies towards more intelligent, efficient, and adaptable financial services.”
Why big data is important for fintech companies?
Financial technologies and big data converge in several key areas, creating a symbiotic relationship that benefits both service providers and consumers. As a result, you can optimise services and streamline customer experiences in the financial sector.
Big data processing has become a crucial tool for fintech companies, enabling them to leverage vast amounts of data to make more informed financial decisions. For example:
- Real-time data processing simplifies monitoring user activities in web and mobile applications, setting the stage for thorough subsequent analysis.
- Data mining dives deep into extensive volumes of unstructured data housed in data lakes, extracting practical insights.
- Data visualisation through adaptable dashboards offers new perspectives on key aspects of core business operations.
Data analytics in fintech companies serves as a key mechanism, transforming massive datasets into valuable insights that drive personalised financial services, risk management, and strategic decision-making.
- Advanced risk analysis algorithms utilise artificial intelligence and machine learning to enhance precision in assessing risks.
- Data-driven decision-making relies on big data analysis and AI/ML algorithms, which guide in determining the best approach in intricate scenarios.
- Automation of business processes, integrated with smart decision-making, minimises service delays and elevates the customer experience.
Data security is not just a regulatory requirement but a cornerstone of customer trust and operational integrity, safeguarding sensitive financial information in the digital world.
- Big data helps to create highly detailed customer profiles, essential for the protection of users’ identities and personal data, which is a critical concern for financial institutions.
- Artificial intelligence and big data are extensively employed to identify unusual activities, preventing diverse forms of fraud and complex hacking endeavours.
These practices, along with other related strategies, constitute the core data management framework for any digital bank or financial technology firm.
The collection of big data and comprehensive 360-degree data analysis has surpassed the status of mere trends in computer science. Instead, they are now fundamental elements for any financial institution aiming to develop and sustain.
How can big data influence the consumer experience in fintech?
Big data in fintech has impacted customer experience in numerous ways. Thanks to it, the financial industry can now.
1. Detect scam faster
Machine learning, along with big data, proved its efficiency in identifying suspicious activities. Yet, these technologies also encounter sophisticated fraud schemes and complex hacking efforts.
For example, Deutsche Bank leverages big data analytics to pinpoint methods used in money laundering, strengthen its know-your-customer protocols, and guard against credit card fraud.
2. Analyse consumer behaviour to offer new services
Since historical financial big data enables companies to track changing customer behaviours, financial institutions can develop products and services that greatly facilitate banking operations.
For example, Oversea-Chinese Banking Corporation (OCBC) devises a highly effective event-based marketing strategy driven by the extensive collection of historical client data.
3. Credit ratings
Financial organisations can provide accurate credit ratings based on critical factors such as the frequency of missed or delayed payments, the total amount of money a client owes, and the swiftness with which they make payments. Such detailed assessments contribute to smarter credit decisions.
4. Client satisfaction survey results
Big data helps understand consumer satisfaction, often drawing insights from survey results. For example, big data is a great tool for managing product and feature requests and analysing trends in consumer support tickets, offering a helicopter view of customer needs and preferences.
5. UI/UX with A/B testing
Big data enables Fintech companies to access real-time information that reveals how users engage with their products, including the average duration spent on their portal, website, or mobile app and which features are most frequently used.
With such data, these companies can compare two product versions to determine which offers a superior UI/UX design. This analysis also provides a deeper understanding of the differences between the products and their impact on the customer experience.
What are the big data use cases in financial services?
Companies, taking advantage of extensive datasets, can extract important insights about customer behaviour and preferences.
It enables them to optimise their operations, cut down on costs, and improve the overall customer experience, leading to more efficient and customer-centric business models. As for now, the most common big data use cases relate to the following.
Customer segmentation
In banking and financial services, big data has several prevalent applications, with customer segmentation being a notable example.
Financial institutions use big data to categorise customers into groups based on their spending behaviours and demographic details. This segmentation leads to highly targeted marketing campaigns tailored to the preferences of specific customer groups.
Fraud detection
By employing AI and machine learning algorithms, banks can proactively identify and flag suspicious activities like money laundering or identity theft, potentially stopping fraud before it occurs.
This is achieved by scrutinising transaction patterns across multiple accounts, thereby enhancing the security and integrity of financial operations.
Risk management
Access to extensive historical market data empowers financial institutions to assess the risks associated with investments or loans more accurately.
These institutions can analyse past market trends using predictive analytics models to forecast future risks and opportunities.
In addition, such a risk assessment approach enables them to make more informed decisions regarding resource allocation towards specific assets or businesses, optimising their investment strategies and financial planning.
Portfolio optimisation
Here, big data provides wider opportunities when creating client portfolios, including strategies for asset allocation, diversification techniques, and projections of expected returns over various time frames. By leveraging big data, portfolio managers can tailor their investment strategies to align closely with individual client goals and risk profiles.
The big data challenges in FinTech
After exploring the benefits of big data in fintech, it’s important to address its challenges.
Regulatory compliance
One can’t deny that the financial sector is subject to strict regulations, especially concerning the storage of customer financial information. Today, privacy concerns are front and centre, so it becomes increasingly challenging for big data FinTech companies to collect and store user data without breaching regulations.
Key regulations such as the Fundamental Review of the Trading Book, Anti-Money Laundering/Know Your Customer, and Foreign Account Tax Compliance Act govern how banking institutions handle user information. Adherence to these and other regulatory standards is a key to big data integration into their operations.
Despite advancements in big data analytics, artificial intelligence, and machine learning, the sector is somewhat impeded by regulations that have not kept pace with technological adoption. This outdated regulatory mindset not only restricts the FinTech sector from adopting more advanced big data analytics solutions and models but also hinders its capacity to better serve user needs.
IT Infrastructure
Another significant challenge, following regulatory concerns, is the IT infrastructure needed for big data in fintech. The implementation of big data in the FinTech sector is still at a nascent stage in many parts of the world. Thus, managing the large amount and variety of data in FinTech while ensuring its quality presents major challenges.
Lacking the right tools and infrastructure makes using and analysing big data difficult, and the integration of AI and ML models into existing organisational systems is even more so. Moreover, most FinTech startups lack the financial resources to immediately incorporate the full spectrum of big data capabilities into their operations. At the same time, larger, more established players often grapple with outdated legacy systems, hindering their ability to fully embrace the advantages of big data.
Data Quality
After infrastructure and regulation, data quality emerges as a critical challenge in the effective use of big data. Simply gathering a lot of data is not useful by itself; the real worth comes from the data’s quality and how companies use it. Yet, many FinTech firms, particularly startups, often overlook the significance of data quality.
This oversight can lead to various errors, such as misdirected product marketing, inaccurate customer profiles, and flawed credit risk assessments. Such mistakes can be costly, not only in terms of losing clients but also in the substantial financial resources required to rectify issues stemming from poor data quality. Additionally, substandard data quality can pose serious risks to regulatory compliance, data security, and the overall reputation of the company.
Final thoughts
Big data is emerging as a perfect tool for reinforcing a user-centric approach in a strong and scalable manner. Its application in FinTech has brought about significant transformations, impacting not just internal company processes but also the customer experience.
This shift in customer experience has become a determinant and a key driving force behind growing customer expectations. Consider this: aren’t FinTech companies outpacing traditional banks by offering what they can’t?
At Altamira, we leverage the latest technologies and expertise in Data Pipelines & MLOps, cloud technologies, user experience design, and artificial intelligence to streamline complex processes and unlock valuable insights into customer behaviour for FinTech companies. The deep customer insights provided by big data yield numerous benefits to businesses, which extend beyond the following key areas:
- Enhanced customer segmentation, allowing for more tailored marketing and service strategies.
- The development of customer-centric services designed to meet specific needs and preferences.
- Improved fraud detection capabilities and strengthened security protocols, ensuring greater protection for both the company and its customers.
- Automated operations, leading to increased efficiency and reduced operational costs.
Contact us to learn how big data help fintech companies to accelerate your growth and become more sustainable by driving digital transformation with big data.
People also asked
Navigating the vast seas of big data in FinTech comes with its set of pitfalls. Firstly, ensuring data privacy and security is a key, given the sensitive nature of financial information. Striking a balance between using data for insights and adhering to stringent regulations like GDPR and CCPA is a tightrope walk. Moreover, the sheer volume and variety of data require sophisticated analytical tools and skilled professionals, which can be a significant investment.
FinTech companies are at the forefront of using big data to stay ahead. By analysing vast amounts of transactional and customer behaviour data, these companies have personalised financial services like never before, offering tailored recommendations and products that meet individual customer needs. Additionally, big data analytics enables FinTech companies to improve risk management through more accurate credit scoring models and fraud detection algorithms.
Big data facilitates risk assessment, where algorithms analyse historical data to forecast future trends and identify potential risks. Big data also streamlines customer service, enabling personalised financial advice and product offerings based on individual spending habits and preferences. Furthermore, it plays a crucial role in detecting and preventing fraud by identifying patterns indicative of fraudulent activity. Lastly, it facilitates algorithmic trading, where high-frequency trading platforms use big data to make split-second decisions on buying or selling stocks.
Through the application of machine learning algorithms and predictive analytics, FinTech companies can unearth deep insights from data, enhancing decision-making processes. Data science also empowers FinTech companies to streamline operations, reduce costs, and detect fraudulent activities with unprecedented accuracy.