Table of Contents
Algorithms have replaced crystal balls, making price forecasting an efficient asset for business strategy and decision-making. Predicting future prices of goods and services enables companies to control risks and maintain a competitive advantage.
Today, AI algorithms can process vast data sets to spot patterns and predict business outcomes.
These algorithms also consider external influences, such as economic indicators, weather conditions, and geopolitical developments, improving the precision of their predictions. Let’s explore the benefits price forecasting can yield for your business.
Understanding price forecasting
Price forecasting uses historical data, statistical methods, and various analytical tools to estimate future prices of specific products, services, or assets.
The goal is to accurately predict future prices, enabling businesses to make strategic decisions regarding production or marketing.
Effective forecasting helps companies anticipate shifts in demand, supply, and overall market dynamics, facilitating risk management, inventory optimisation, and resource management.
Gartner`s Tech Trends 2021: What is Special about Total Experience?
AI forecasting model: key concepts and applications
AI forecasting uses machine learning algorithms to predict future events by analyzing vast datasets. Unlike traditional forecasting methods, which rely on fixed statistical models, AI forecasting can identify hidden patterns and trends in both structured and unstructured data. This capability makes it an essential tool in various industries, from finance to supply chain management, where accurate demand sensing and risk assessment are crucial.
Advantages of AI forecasting over traditional methods
AI forecasting offers several advantages over traditional approaches. By leveraging advanced machine learning techniques, it can handle complex, non-linear data patterns that conventional models struggle with. Additionally, AI forecasting is fully data-driven, reducing the impact of human bias and improving overall accuracy. It also automates data processing and modelling, making forecasting more efficient and significantly reducing cycle time.
Data handling in AI forecasting: structured and unstructured data
One of AI forecasting’s key strengths is its ability to process both structured and unstructured data. Structured data, such as sales figures and financial records, can be analyzed alongside unstructured data, such as social media trends or customer reviews. This broader data integration allows businesses to gain deeper insights into market behavior and future demand trends.
AI forecasting tools and technologies
AI forecasting relies on cutting-edge machine learning models that continuously refine their predictions as new data becomes available. These tools can forecast in real time, delivering instant insights that support fast decision-making and operational adjustments. Scalable architectures ensure that businesses can expand their forecasting capabilities as their datasets grow.
Advanced algorithms and techniques in AI forecasting
The power of AI forecasting comes from its ability to uncover complex relationships in large datasets. Deep learning, neural networks, and statistical machine learning models help detect trends that traditional methods might overlook. These techniques also enable AI forecasting to connect historical data with real-time inputs, making it an effective tool for demand sensing and financial predictions.
Improving prediction accuracy with AI Forecasting
AI forecasting enhances prediction accuracy by analyzing large volumes of data and eliminating manual errors. It can detect subtle shifts in demand, revenue, and operational patterns, helping businesses make data-driven decisions with greater confidence. This leads to more reliable revenue forecasting, expense management, and risk assessment.
Real-time forecasting and decision-making with AI
One of AI forecasting’s standout features is its ability to provide real-time insights. Businesses can respond quickly to market fluctuations, supply chain disruptions, or financial risks by continuously updating forecasts with the latest data. This agility offers a significant competitive advantage, enabling organizations to adapt to changing conditions more effectively.
Scalability and efficiency in AI forecasting
AI forecasting models are highly scalable, making them suitable for businesses of all sizes. As datasets grow, AI-driven forecasting tools can seamlessly handle increased data loads while maintaining speed and accuracy. This efficiency improves forecasting quality and reduces operational costs by optimizing resource allocation and minimizing risk.
AI forecasting in demand planning and supply chain management
AI forecasting is a key tool in modern supply chain management, helping companies transition from traditional forecasting methods to true demand sensing. By integrating historical sales data, real-time market trends, and predictive analytics, AI forecasting enhances inventory management, production planning, and logistics efficiency.
Challenges and considerations in implementing AI forecasting
While AI forecasting offers numerous benefits, it also comes with challenges. Successful implementation requires large datasets, skilled data scientists, and ongoing model refinement. Additionally, businesses must consider data privacy, integration complexities, and computational costs. Despite these hurdles, the growing need for accurate, automated forecasting solutions continues to drive AI adoption across industries.
This structured approach highlights AI forecasting’s core benefits, applications, and challenges, ensuring businesses understand its potential impact on decision-making and operational efficiency.
Descriptive and predictive analytics
Descriptive analytics employs statistical methods for data collection, analysis, interpretation, and presentation. This approach transforms raw data into understandable knowledge, answering the question, “What happened?”.
On the other hand, predictive analytics focuses on using current and historical data to envision future events, outcomes, or values, particularly in price forecasting.
It involves various statistical techniques to identify data patterns, including data mining and machine learning.
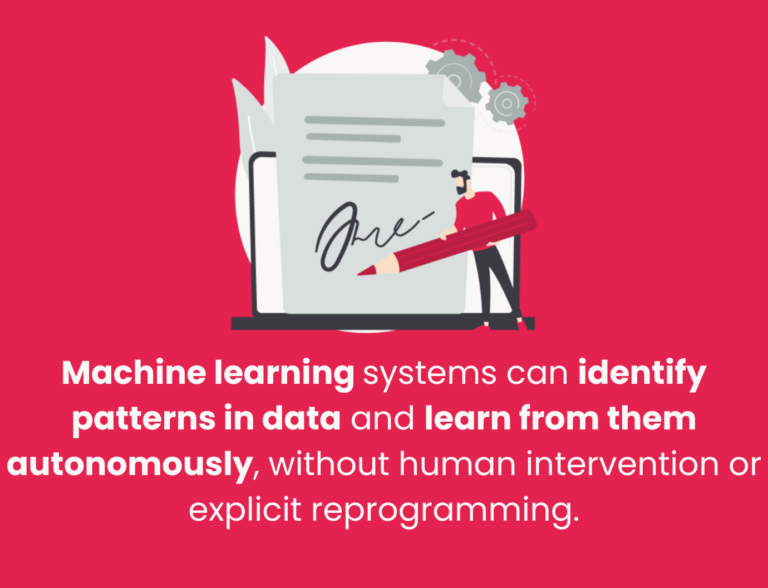
Thus, data scientists first determine the relevant data for training price forecasting machine learning models, where descriptive analytics plays an integral role.
AI adoption framework: technology/privacy/ security/data point of view and EU AI act
The role of AI and Machine Learning in price forecasting
Artificial intelligence and machine learning offer businesses, regardless of size, extended capabilities by analysing and managing extensive datasets. This transformative impact naturally leads us to examine the specific benefits that AI and ML bring to the domain of price forecasting:
Pattern recognition: ML algorithms excel at identifying patterns and correlations in data that might not be obvious to human analysts. This can include seasonal trends, price elasticity, and consumer purchasing behaviours.
Real-time analysis: AI systems provide real-time analysis, which is important for dynamic pricing strategies in industries like retail, where prices may need to change rapidly in response to market conditions.
Industry-specific price forecasting: AI and ML models can be tailored to specific industries or products, allowing for more relevant price forecasting.
Market sentiment analysis: AI efficiently analyzes news, social media, and other textual data using techniques like natural language processing to gauge market sentiment, which can influence price movements.
Continuous learning: ML models learn from new data and outcomes, constantly improving and refining their forecasting accuracy over time.
Error reduction: Automating the price prediction process reduces the scope for human error and bias. It also saves considerable time and effort previously spent on manual data analysis and forecasting.
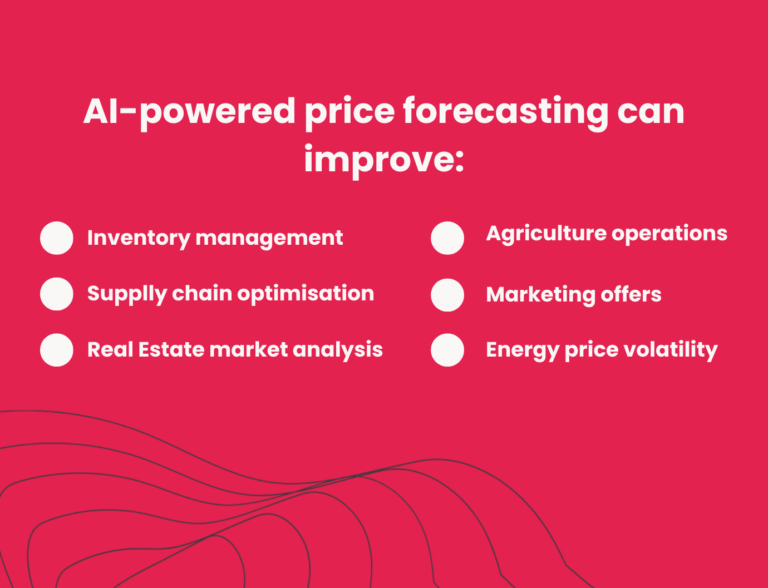
Overall, AI and ML transform price forecasting into a more data-driven, precise, and efficient process, enabling businesses to make more informed decisions based on comprehensive market analysis.
See also: Real Estate Digital Transformation in 2022: New Trends You Should Adopt
What industries benefit the most from price prediction?
Price prediction models, fuelled by artificial intelligence, have become integral to the pricing strategies of numerous domains, including the following.
Travel industry
The travel industry, particularly airlines and hotels, is reaping significant benefits from artificial intelligence-powered price prediction.
By employing machine learning algorithms, these businesses accurately project future pricing trends.
With the help of machine learning models, companies use historical data and competitor pricing information, ensuring their price estimates are well-founded and competitive.
Creating competitive prices for seats or rooms that align with market sentiment can profoundly impact sales volume and profit margins.
The real-time outputs machine learning algorithms provide ensure that these businesses consistently stay competitive.
Retail
Price prediction is equally vital in the retail industry. Retailers widely use price prediction models to set competitive prices with other stores, helping keep their products appealing.
Retailers can further employ these models to forecast customer satisfaction at various price points, enabling them to offer targeted discounts or coupons for specific items.
Machine learning models also can anticipate pricing trends over time, allowing retailers to adjust their strategies and thus optimise profits.
Predicting stock prices using AI
The stock market forecasting is characterised by its volatility and dynamism. Price forecasting becomes difficult due to the influence of numerous macro and micro factors, including political dynamics, global economic conditions, unforeseen events, and a company’s financial performance, among others.
The interest in employing machine learning for stock prediction is more than just a trend; concrete outcomes support it.
For example, the AI-powered hedge funds generated average monthly returns of about 0.75%, compared to about 0.25% for the human-guided hedge funds.
This clearly demonstrates that using AI for stock prediction is crucial for distinguishing between capitalizing on an opportunity and missing it in the rapidly changing financial landscape, where fortunes can be made or lost in seconds.
Data requirements for price forecasting
Every day, we generate approximately 328.77 million terabytes of data, a number that continues to grow due to our increasingly interconnected activities driven by global digitalization.
Big data ecosystems excel in capturing, storing, and managing these vast quantities of data, laying the groundwork for insightful analysis and value extraction.
Thus, businesses proactively use data to optimise their operations, reduce expenses, and boost profits.
However, data management is critically important for any business as it ensures the efficient and secure storage, organization, and retrieval of data, enabling informed decision-making and business operations.
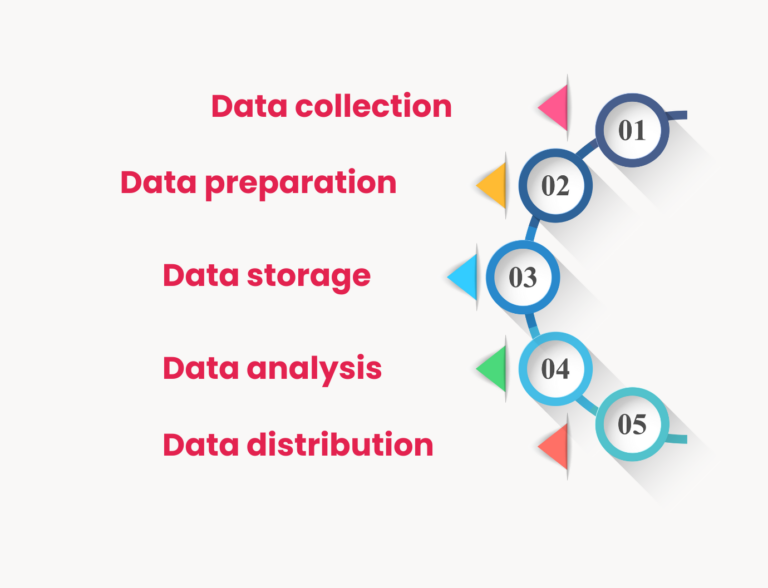
The success of AI-powered solutions heavily relies on the quality of data they are trained and operate on. Poor data quality can lead to errors, biases, and unreliable outcomes. Improving data quality involves:
Establishing clear data governance policies
Conducting a data quality assessment
Data standardization and validation
Data cleansing
Implementing robust data integration
Monitoring data quality
Continuous improvement
Ample data can enable the training of more complex models. Yet, acquiring more data also increases the time, costs, and resources needed for its collection, storage, processing, and analysis.
Generally, more data results in more dependable models and improved outcomes, given the data is authentic and representative. It’s better to use a smaller amount of high-quality data than a larger volume of poor-quality data.
The bottom line
As the volume of data generated continues to grow, AI will increasingly become a valuable asset for businesses seeking to maintain a competitive edge. By using AI and historical pricing data, companies can reveal insights beyond human capacity, enabling more informed decisions.
We can also now experiment with various scenarios and identify the most profitable price points, considering changes in costs, inventory, and pricing.
However, adopting new technologies is not enough. First, companies should implement a framework of procedures to regulate data management to ensure the effectiveness of advanced analytics and AI solutions.
At Altamira, we provide Data Governance, Data Pipelines, and MLOps services to help you prioritize and establish the proper data governance practices needed for efficient price forecasting.
We also help businesses estimate market volatility, manage risks, and optimise their business strategies to steer dynamic markets with precision and confidence.
Predictive analytics is a new must, whether for stock price prediction using machine learning, energy resource optimization, or weather forecasting. Learn how your business can benefit from AI-powered price forecasting!
FAQ
An AI forecasting model consists of several essential components, including data collection, preprocessing, feature selection, model training, and evaluation. The process begins with gathering historical and real-time data, which is then cleaned and structured for analysis. The model is trained using machine learning algorithms, and its performance is fine-tuned based on accuracy metrics before being deployed for predictions.
AI models are only as good as the data they analyze. Data cleaning and validation ensure accuracy by removing errors, inconsistencies, and missing values. Poor-quality data can lead to misleading predictions, while well-prepared datasets allow the model to detect reliable patterns and trends.
This step also ensures that forecasts remain consistent across different time frames and datasets.
Time series data is crucial for AI forecasting because it captures patterns, trends, and seasonality over time. By analyzing past data points in sequence, AI models can recognize recurring trends, anomalies, and sudden shifts, improving the reliability of future predictions. This is especially useful in fields like financial forecasting, demand planning, and weather prediction.
AI forecasting leverages machine learning algorithms, statistical modelling, and deep learning techniques to analyze complex data. Methods such as regression analysis, neural networks, decision trees, and reinforcement learning help the model adapt to non-linear relationships and hidden dependencies in datasets. These approaches allow AI to process both structured and unstructured data, providing deeper insights than traditional forecasting methods.
Machine learning enhances forecasting accuracy by automating data analysis, identifying intricate patterns, and adjusting predictions based on new data. Unlike traditional models, which rely on predefined equations, machine learning algorithms can continuously learn from past mistakes and refine their predictions. This dynamic approach leads to more precise and adaptable forecasts across industries.
Deep learning, a subset of machine learning, uses neural networks to recognize highly complex patterns in large datasets. It excels in analyzing high-dimensional data, unstructured text, and time-dependent trends. Deep learning models, such as Long Short-Term Memory (LSTM) networks and Transformers, are particularly effective for forecasting financial trends, demand fluctuations, and operational risks, making AI predictions more accurate and actionable.