Table of Contents
Global investment in AI systems reached 184 billion U.S. dollars in 2024, a considerable jump of nearly 50 billion compared to the previous year. This wave of interest has led businesses worldwide to ask the only question: What are the costs of implementing AI?
Unfortunately, there isn’t a one-size-fits-all answer. The development and further support of a custom intelligent system depend on numerous factors, making the cost of AI projects uniquely estimated for each case. So, let’s shed light on the key cost drivers impacting AI projects and provide approximate sum to give a general sense of AI costs.
The rise of AI development in business
The need for automation floats around and has never been more important. As a result, leading companies more and more often rely on AI-powered systems to maintain business resilience by all means. From optimising inventory to transforming customer service, artificial intelligence offers striking opportunities to automate workflows and yield whopping cost savings. Thus, artificial intelligence adoption brings to the table a wealth of benefits.
Data management
Artificial Intelligence empowers organisations to take advantage of incoming data, e.g., AI technology can monitor the company’s communication channels, thereby reducing the risk of missed customer requests or low employee performance.
Predictive analytics
AI systems allow businesses to identify hidden trends, patterns, and dependencies within their data, leading to improved forecasting. Predictive analytics also provides opportunities for selling follow-up products and services, optimises inventory, and enhances daily operations.
Reduced manual effort
It is clear without saying that by automating business processes, companies can speed up workflows. Think of automating tasks like email distribution, customer service with chatbots, order processing, and other everyday activities that consume time.
The rocky road to AI adoption
You have to admit that the price of AI adoption is heavily impacted by the scope of the envisioned AI-powered system. Successful implementation of AI technology aligned with relevant business goals and needs requires any business to overcome several challenges. Let’s explore it in more detail.
Inaccurate data
The AI implementation process relies on the right dataset with complete and accurate data. No matter if it’s labelled or unlabelled data, you will definitely need a data repository to train efficient models.
At the same time, choosing the right dataset can be quite a challenge, especially for those grappling with vast amounts of unstructured data scattered across various systems. To tackle this issue, many organisations turn to AI professionals for guidance in determining the most relevant and qualitative datasets for the upcoming project.
At the crossroads of technology unknowns, Altamira helps you build AI roadmap, maintain data quality, and develop feature-rich, fine-tuned solutions tailored to automate and improve your manual business operations.
AI integration into existing systems
Enterprises are most likely to seek AI adoption. However, integrating artificial intelligence with existing systems can be no small feat. This challenge arises from the need to gather, clean, and unify data into a single source of truth.
Algorithms and model training
As you know, training a model always requires a lot of data, and it definitely takes some time. Additionally, to translate business intelligence into real-world predictions, your model must be deployed in production. What’s more important to keep in mind is that AI algorithms require continuous training and enough high-quality data to remain accurate.
AI software development cost factors
Like other tech projects, AI pricing follows basic estimation principles with some unique modifications. Here are some factors that greatly affect the costs of AI projects.
Cooperation model
The approach to AI development you choose impacts the cost:
- Outstaffing: Engaging an external vendor offers niche expertise in the AI domain, qualified resources, and access to advanced technologies, which can accelerate project timelines and enhance the overall quality of AI implementations.
- In-house software development: Despite the fact that building an internal AI team gives you more control over the development process, this initiative requires substantial investments in sourcing, recruiting, training, and retaining highly skilled AI engineers.
- Hybrid model: Combining in-house development process with outstaffing enables you to use internal knowledge while compensating skill and knowledge gaps with vendor’s resources.
Hire dedicated software development teams to build effective solutions, scale your business, and achieve lasting growth.
AI solution functionality
The list of features you want in the AI-powered software greatly affects the project’s cost. Custom-made AI solutions designed to meet specific needs typically incur higher development costs compared to off-the-shelf options, which are faster to implement and potentially cheaper but may not fully comply with your business requirements.
Project type
The cost of your AI project is always influenced by its complexity. Contributing factors include:
- Data quality and volume: Your data appears to be the key factor in building a dependable AI model. Keep in mind that challenges such as missing and unstructured data, on top of the need for thorough data cleaning, can escalate costs.
- Algorithm performance: The expected level of accuracy of your AI algorithm greatly impacts costs. For example, developing an image recognition system that requires a high accuracy level will be more expensive than creating an application with greater room for error.
Cost estimation calculator
Explore our new cost estimation calculator designed to empower our clients with quick and accurate assessments of software development project costs.
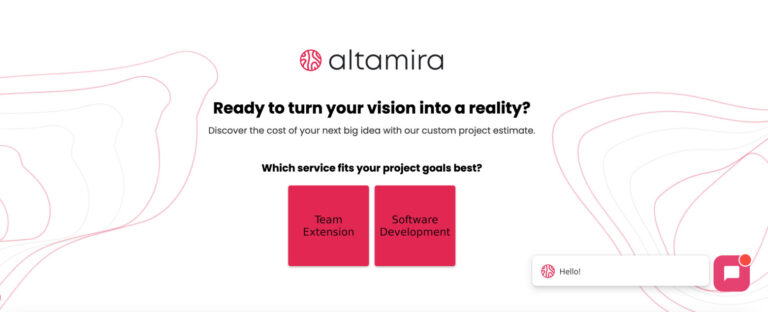
This tool streamlines the initial planning phase, providing transparency and clarity to help you make informed decisions about your project budget.
Whether you’re envisioning a custom AI solution or a mobile app, our calculator ensures you start off on the right foot with a clear understanding of financial expectations.
Stages of AI implementation: from concept to deployment
Creating a highly functional solution, such as AI-powered software, involves a gradual and iterative process, which unfolds in multiple phases – each requiring financial and human resources.
Understanding how AI projects are budgeted at every stage of development is a must, especially if you are considering an outstaffing option. The sequence and duration of these stages can vary depending on the scope and specific AI features you aim to incorporate into your product.
AI feasibility study
Many AI initiatives require initial research and development efforts to determine the optimal delivery approach. So, initiating AI deployment often implies starting with a Discovery phase or Proof of Concept.
Typically, this initial phase requires a budget ranging from $15,000 to $30,000 in over 90% of cases. This initial investment, often referred to as seed capital, helps validate your concept early on and clarify the final product or solution. It also helps mitigate risks of project failure and can potentially lead to long-term cost savings.
For the development team, this stage helps to identify the most suitable technical approach and the most cost-effective solution tailored to your specific requirements. Conducting feasibility studies also allows vendors to ensure the availability and adequacy of necessary data, which is key to the success of AI projects.
Here are some of the outcomes you can anticipate from this stage of development:
- Problem Analysis: The development journey begins with a 360-degree overview of your business requirements. The goal is to align project objectives with the technical capabilities of your AI engineering team. So, the project stakeholders have to clearly define the business challenge you aim to address.
The more detailed your specifications are, the more effective your AI implementation is likely to be. The cost of this stage may vary depending on the problem’s difficulty. - Detailed Business Analysis: A thorough business analysis is a must for AI engineers to grasp the project’s mission. By clearly understanding the goals, developers can align your business requirements with the most suitable technical solutions. This analysis should result in outlined functional and non-functional requirements for the AI system, covering aspects such as accuracy, privacy, and response time.
Architecture design
Here are some of the expected outcomes from this development stage.
Again, the duration of this phase heavily depends on how complex your software is. Solution architecture serves as a bridge between your business challenges and the technological solutions needed to solve them. This stage covers:
- The identification of the most suitable tech stack to tackle your business challenge.
- The description of the structure, features, behaviour, and other elements of the solution to stakeholders.
- The selection of suitable integration methods and processes.
Software development
In this phase, the AI implementation work is in full swing. Within AI development, it can be segmented into two primary phases:
- Front-end and back-end development: Initially, the AI team creates the software’s front-end user interface and back-end logic, incorporating APIs for the AI components.
- AI module development: This phase is focused on preparing data for the machine learning algorithm, which consumes the most time in AI development and greatly impacts overall costs. Here’s a detailed breakdown of this phase:
- Data preparation: ML engineers assess the adequacy of available data for the project. If the data is in tabular form, they visualise it for better comprehension and cleanse it to eliminate duplicates.
- Data collection (when necessary): In the perfect world, all required data is available internally. However, if data diversity is lacking or specific data is missing, additional collection efforts are required. Raw data may need preprocessing to enhance model training efficiency.
- Baseline model creation: Once all data is gathered, data engineers develop a baseline model as an initial benchmark. Baseline models are easy to set up, cost-effective, and rapidly provide initial insights. They also serve as a basis for predicting the performance of more complex models.
After confirming the quality of the baseline model, the team proceeds to develop more advanced machine learning models. Prototyping and creating Minimum Viable Products are also common practices for validating and iterating on the solution further.
ML model training
As a rule, this phase spans several weeks, depending upon the model’s complexity. During this stage, you can see the following:
- ML engineers carefully choose the most appropriate machine learning algorithms to construct the model.
- The selected algorithms are trained using specific datasets and then tested against separate samples. Engineers validate the final model in a pre-production environment using a test dataset.
Deployment
Finally, the solution is ready for deployment. Vendors often suggest a pilot deployment to a small user group to ensure a smooth rollout. This pilot phase confirms compatibility with existing infrastructure and allows for user acceptance testing to resolve any issues before a full-scale launch.
Maintenance
Software maintenance is just as essential as software development, so don’t neglect it and include maintenance costs in your AI budget. The development team will oversee the performance of the deployed solution. Regular adjustments and retraining of AI components are common, driven by ongoing data updates. Additionally, if your AI solution is for public use, budgeting for marketing efforts is also a must.
Case study spotlight
Elula
Altamira team delivered an AI-powered solution for children’s academic success empowerment.
Elula, a forward-thinking technology company based in the US, is dedicated to revolutionising education by empowering children to enhance their confidence, skills, and joy of learning. They provide innovative solutions aimed at boosting academic performance and fostering stronger connections between children and their parents to overcome learning barriers.
In response to the growing demand for accessible neuropsychological assessments, Altamira introduced Uluru, a transformative AI-powered solution. This platform revolutionises the traditional approach to diagnosing and monitoring cognitive, mood, and personality disorders in children, offering a more efficient and stress-free alternative to conventional methods.
The implementation of Uluru involved several phases:
- Discovery Stage: Research and planning to identify key requirements and goals.
- Web and Mobile MVP Development: Building the MVP for web and mobile platforms to ensure accessibility.
- Integration of AI Features: Incorporating advanced AI capabilities to streamline and expedite the diagnostic process.
- Maintenance and Continuous Improvement: Ongoing updates and enhancements to optimise performance and user experience.
Key features of Uluru:
- Neuropsych Testing: Using AI and advanced analytics for quick and impartial assessments of cognitive functions and continuous monitoring.
- Personalised Coaching: Generating tailored recommendations based on assessment outcomes to enhance executive functions critical for learning and daily life.
- Learning Strategies: Providing effective learning techniques to improve educational outcomes for students.
- Integration with LMS Platforms: Initially compatible with Google Classroom, with future plans for integration with other major Learning Management Systems (LMS) like Schoology, Blackbaud, Black Board, Infinite Campus, and Canvas.
- Parent Dashboard: Offering insights into a child’s progress and personalised coaching suggestions for parents to support their child’s learning journey.
Product detection solution for retail company
Altamira successfully completed a Proof-of-Concept development for an AI-powered solution aimed at automating processes and optimising workflows in retail environments. The solution, designed for a global retail company operating in the USA, Spain, Central America, Brazil, Colombia, Chile, Argentina, Peru, and India, focuses on streamlining staff workflows within stores.
The Client sought to enhance operational efficiency by optimising how staff manage shelf inventory and product placement across multiple stores worldwide.
Altamira presented the client with a scalable smart data capture and retail shelf analytics solution. This solution leverages AI and real-time data processing to streamline inventory management directly from staff mobile devices.
Key features:
- Real-time Image Capture: The application continuously captures shelf images as staff move their devices, eliminating the need for manual photo input.
- Automated Object Recognition: Powered by AI, the app detects and outlines products on the shelves in real time using bounding boxes.
- Confidence Scoring: Each identified product comes with a confidence score, indicating the AI’s certainty in its identification.
- Instant Analytical Feedback: Users can command the app to process and display recognised items with their quantities immediately.
- Cloud Integration: The app communicates with a cloud-based system to match detected items with an up-to-date product database for accurate information retrieval and storage.
- Inventory List: After analysis, the app generates a concise inventory list detailing product names, counts, and other relevant details.
- User Interaction: Featuring an intuitive interface, users simply press an “Analyse” button to finalise the scanning process and review the inventory count.
- API Communication: For product recognition, the app integrates with APIs to send data to the cloud for detailed analysis.
- Dynamic Result Display: The final inventory count overlays on the live camera feed, allowing staff to cross-reference digital data with physical products in real time.
Strategic partnerships: key to successful AI implementation
Implementing AI can be quite a complicated and costly task, underscoring the importance of selecting a seasoned vendor. At Altamira AI, we understand both the substantial challenges posed by AI adoption and its huge potential. That’s why we developed the AI Adoption Framework, in order not to lose the wave of booming artificial intelligence capabilities but to adopt best practices allowing you to integrate AI/ML solutions seamlessly.
What areas does the AI adoption framework cover?
- Data quality, availability, accessibility, and management
- Data protection and privacy
- Legal and regulatory obligations (EU AI Act)
- Ethical concerns and risk management
- Effectivity improvement
- Cost effectivity, sustainability, and total cost of ownership
Our team of AI experts guides partners through every step of the AI adoption journey, ensuring tangible outcomes and maximising their investments. Get in touch to get a free expert consultation!