Table of Contents
For the last 10 years, artificial intelligence has been one of the discussed technologies. However, the history of AI development started almost a century ago.
Scientists have been working to build a machine with human-like intelligence since the 50s, and with current technologies, we have finally seen substantial progress.
Nowadays, artificial intelligence (AI) is almost everywhere. From a regular user’s perspective, it’s a fast and efficient tool that helps with daily routine and business needs. But under this simple idea lies a complex system with hundreds of specific technologies aiming to provide fast and efficient results.
What is artificial intelligence?
Artificial intelligence is a field of computer science focused on creating systems that can simulate aspects of human intelligence, including learning from data, recognising patterns, and solving problems.
To achieve human-like behaviour, the AI has to be able to:
- Learn;
- Make independent decisions;
- Recognise patterns.
There are many interpretations of what AI is, but the outlining idea always stays the same. One of the AI pioneers, CEO of Microsoft AI Mustafa Suleyman has recently given his own take on what AI is and what we should expect from it in the future:
Despite being in the works for almost 75 years, the real breakthrough in AI development happened in the last decade. Our technological advancements in computing have given us tools that can learn from given data, however, we still remain far from conscious AI systems.
Contrary to popular belief, AI is not a “magic wand” that can solve any problem. It’s a powerful tool, but it has its limits. Functional types of AI describe these limits and help businesses understand what to expect.
See also: Implementing AI: Is it worth it? A step-by-step guide to AI implementation
Functional types of AI
For some businesses, AI might seem like a mysterious tool that can do anything. The topic often feels too complex to grasp, but adopting the technology business knows nothing about might lead to unfortunate results. As Altamira’s Chief Delivery Officer, Natalie Semak, said recently:
“When you remove the mystery from AI, leaders make better decisions. They stop chasing trends and focus on practical applications that drive real business value. The result? Clear, actionable plans that align technology with business objectives. Technology should solve problems, not create them. Sometimes the best innovations come from making complex things simple.”
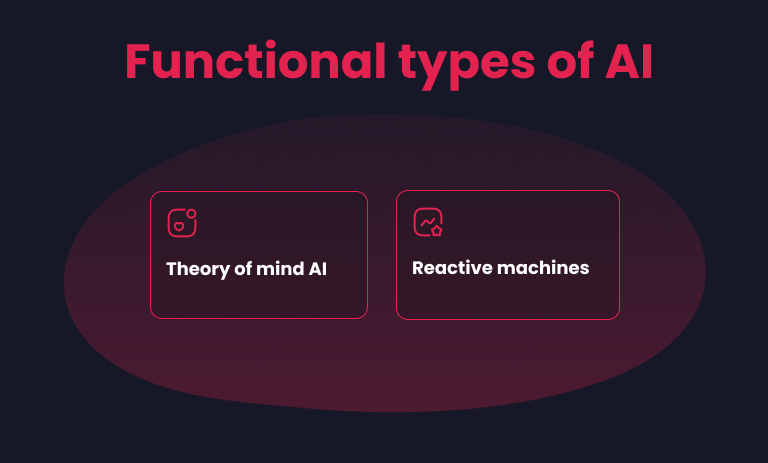
Reactive machines
As the simplest form of AI, reactive machines can only respond to specific inputs with pre-programmed outputs. Simply put, reactive machines can’t do anything outside of known answers.
Such machines can’t learn new information or adapt their responses — they only follow strict rules. A good example of a reactive machine is IBM’s chess bot, Deep Blue.
Released in 1997, Deep Blue was the world’s first computer to win a chess match against the world champion. At its core, Deep Blue could evaluate millions of possible positions in a matter of seconds to make the statistically best move. However, it didn’t have a memory of its previous matches, so it couldn’t adapt or improve.
Limited memory AI
When talking about modern versions of AI, most of them fall under this category. Limited memory AI can learn and make decisions based on the given information. Unlike reactive machines, this type of AI isn’t rule-based; it’s more flexible and can adapt to a changing environment.
Most limited memory AIs have a restriction; they can remember only recent information, so there are no long-term memories.
The automotive industry gave us one of the most well-known examples of a limited memory AI: self-driving cars.
They can detect nearby cars, pedestrians, or other obstacles, but they can’t remember them. The only information self-driving cars store in their memory is the last few seconds. While it might seem like a drawback, in reality, it’s more than enough information for AI to analyse the situation on the road and make decisions.
To sum up, artificial intelligence nowadays can complete truly impressive tasks. However, the true power of AI is in the technologies.
See also: The rise of AI-native technologies
Technological types of AI systems and their applications
When talking about the AI’s variety, it’s also important to point out how it handles business tasks. The technologies behind AI vary, but the most prominent ones are:
Machine Learning (ML)
Machine learning is a subset of AI in which systems learn from data. The core idea is that algorithms can make predictions or decisions based on past examples instead of being explicitly programmed for every scenario.
ML algorithms are behind the recommendation engines at Amazon and Netflix. They analyse user taste, browsing, and shopping history. On top of that, ML algorithms also enhance personalised user experience by identifying offers that align with user interests. ML also enables monitoring campaigns through metrics like open and click-through rates.
ML are highly adaptable and efficient, but the main benefit is in how it can be applied. These models could be used for practically any industry, from commerce to healthcare.
It also has some limitations. The ML model will be as good as the training data, meaning that if the data contains biased or incorrect information, it will lead to faulty results.
Deep learning
As part of machine learning, deep learning can solve more complex problems using neural networks. These networks were inspired by human brains, meaning they are also complex and multilayered. Deep learning models can process large volumes of data and are a perfect choice for finding patterns in unlabelled data.
One of the examples of how deep learning is applied in real life comes from Google. One of their deep learning models could detect early signs of diabetic eye disease.
These models are very accurate and scalable but have the same dependency on data as ML models.
Natural Language Processing (NLP)
This type of AI aims to make machines understand and generate human speech, both written and spoken. Nowadays, almost every spellcheck, translation or speech recognition tool uses NLP.
It is also the technology behind real-time translation apps, voice assistants, and some chatbots. While it still has its shortcomings (inability to understand nuances, like sarcasm or idioms), it’s one of the most widely used technologies.
Computer vision
Another technology that helps machines perceive the real world is computer vision. It enables machines to see, interpret, and make sense of visual data, like photos, videos, or live camera feeds.
This is the technology behind image recognition software that enhances security, detects defects, and, with proper training, recognises people.
Once trained, computer vision technology can process any media content in seconds, and it’s more accurate in spotting anomalies compared to humans.
However, it is sensitive to the quality and angles. For example, if the training data only includes frontal pictures of faces, computer vision might have trouble recognising the same person’s photo, but from a different angle.
Robotics
Robotics bridge the gap between the digital and physical worlds, enabling AI to interact with real-world objects. While not all robots use AI, the ones that do are capable of tasks like navigation, object manipulation, and interaction with humans.
One of the most well-known examples of AI-powered robotics comes from Boston Dynamics’ robot, Spot. It can perform tasks such as inspections, carrying payloads, and allows for both remote control and fully autonomous work.
Despite the idea of robotic workers sounding promising, it’s not cheap to make. Additionally, it comes with some drawbacks, such as limited decision-making in unknown environments and higher energy dependence compared to non-AI robots.
Expert systems
An expert system is a type of AI designed to mimic human-like decisions in a narrow, well-defined area. These systems don’t learn or adapt like machine learning models — instead, they follow a set of predefined rules and logic.
Expert systems work best as decision support tools in the area. For example, CaDet (Cancer Decision Support Tool), analyses patient’s personal information to detect early signs of cancer. Based on CaDet’s evaluation, doctors could advise appropriate measures for cancer prevention.
Expert systems are perfect decision-makers for the types of jobs that require precision and consistency. They can’t recognise patterns, making them very similar to reactive machines, but there’s a difference.
Unlike reactive machines, expert systems heavily rely on their memory, even if they can’t learn new information.
How AI solves business problems
AI applications for business vary depending on the technologies behind it. For example, according to Statista, the global Machine Learning market is projected to reach $105.45bn USD by the end of the year. At the same time, Computer Vision market is projected to reach $29.88bn USD in 2025.
The global AI adoption reinforces the idea of artificial intelligence being the next big step in business efficiency, and there’s a good reason for it. Take a look at how AI solves business problems:
Improve customer service
Using ML and deep learning models with NLP, businesses can build AI-powered chatbot or virtual assistant. Unlike humans, who are prone to making mistakes, AI-powered chatbots perfectly handle routine questions.
Predictive analytics
A lot of businesses, especially in retail or finance, rely on predicting future trends. These industries constantly deal with huge data flows that humans can’t properly process by themselves. Luckily, AI models can process information in a matter of seconds and leave no room for human error.
AI can also find hidden patterns and make more accurate predictions compared to humans.
Fraud detection and cybersecurity
Since most AI learns from data to recognise patterns, it’s also helpful to detect potential fraud or cyberattacks. AI-powered security systems can monitor thousands of transactions and notice potential cyber threats before they happen.
Supply chain optimisation
AI helps businesses make smarter decisions about how goods are sourced, manufactured, and delivered. AI systems can anticipate potential bottlenecks and point out cost-saving opportunities for real-time data analysis of suppliers, warehouses, and delivery fleets. This leads to fewer shortages, less waste, and better overall coordination.
Talent management
In HR, AI can screen resumes, identify patterns in employee performance, and even suggest training paths. While not perfect, it adds another layer of insight to the hiring and development process.
See also: How generative AI is transforming EdTech
Final words
AI is a tool that brings efficiency and automation to every industry. Everywhere you go, there’s a chance you will encounter an AI-powered app or chatbot to help you.
Despite being so widespread, there’s still a lot of confusion about what AI is and how it works. Understanding the inner workings of AI and how it’s applied can help cut through the noise and find a best-fitting AI-powered solution for your business needs.
How Altamira can help
If you’re interested in adding AI-powered tools to your business, need consultation, or want to bring automation to your workflow, our approach guarantees tangible results.
Stress-free development: Build your AI-powered products with a team of experts following best industry practices;
Zero-headaches software partner: Get tangible value in a matter of days, not weeks — we start working on your project even before the contract begins;
Transparent communication: Always be in the loop and know everything that’s going on with your project on a daily or weekly basis.
Get in touch and start building your AI-powered products today.
FAQ about AI deep dive
The deep dive method is a focused approach to learning or problem-solving where you deliberately narrow in on one topic, process, or issue to fully understand it.
Instead of skimming the surface or multitasking across multiple areas, you stay with one area long enough to uncover details, patterns, and root causes that might otherwise go unnoticed.
Deep diving gives you the space to explore the “why” and “how” behind a subject. It’s often used in research, product development, education, or troubleshooting.
The goal isn’t just to collect information—it’s to gain clarity and insight that you can actually apply. Done well, a deep dive can expose what’s working, what’s not, and what to do next.
Deep AI generally refers to artificial intelligence systems that rely on deep learning—neural networks with many layers that learn from large amounts of data.
This is the type of AI behind things like image recognition, language translation, and generative models. It’s called “deep” because of the structure of the learning models, not because it’s more profound in a philosophical sense.